Building explainable AI
- Usage of AI does not come free
- Employ AI to enhance customer experience
- Building explainable AI is far from trivial
This year’s edition of AI for Finance was organized as a 100 percent digital experience. The conference is a platform that brings together professionals from banking and insurance companies, suppliers, and (data) scientists to discuss the developments in artificial intelligence (AI), and in digital transformation in general. CFO Services’ Expert manager Benjamin De Leeuw attended the conference. In this first article in a series of three, he discusses topics on AI that were discussed in the International Stage conference track.
Upscaling AI
With last year’s edition prompting for action, the main focus this year was the ‘passage à l’échelle’, upscaling AI. Conference speakers, mostly from banking and insurance companies, talked about how they employed AI and how their organizations have realized the passage à l’échelle so far.
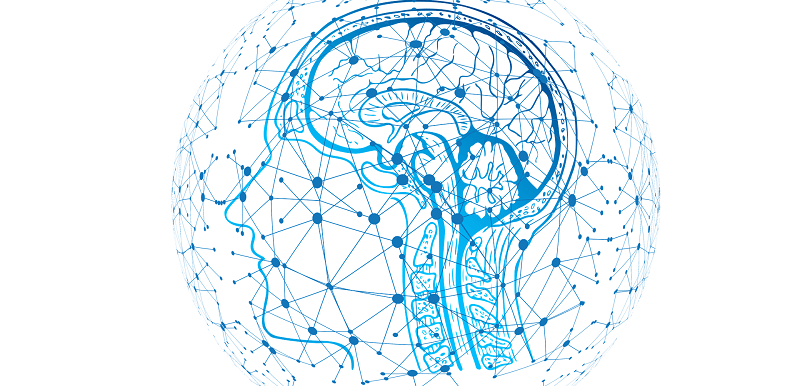
‘DataOps’ as best-practice
Looking around, we see the digital transformation of companies accelerating, mostly driven by technological advances. Companies and their customers generate huge data streams through many different channels: social media, the Internet of Things, online banking, etc.
The goals of digital transformation are manifold. One goal is to retain and analyze the data streams that pass through a company. Another, to predict risks and avoid them. A third, to spot opportunities for enhanced or new products in a fashion that is compliant with regulations.
Scientists estimate that over 80 percent of Cloud data has been generated over the past two years only, putting enormous pressure on analysts to keep up. For banks that are globally active, quantities of data go up to 200 petabytes (10 bytes to the power of 15). Because of their position as a trusted partner, banks and insurance companies have access to even more data.
Analyzing the available data in its entirety, however, is becoming too complex for humans. That’s why companies can reap huge benefits from employing AI technology to help humans analyze and understand this data. a
Scientists estimate that over 80 percent of Cloud data has been generated over the past two years only, putting enormous pressure on analysts to keep up.
Benjamin De Leeuw
Usage of AI does not come free
Companies must rethink and reinvent their processes. Employees might be in need of re-education to become more data-savvy, and the data itself needs to be managed. Hinting at the software development best-practices paradigm ‘DevOps’, conference speakers regularly used ‘DataOps’ to name data management best practices. These include that data is
- cleaned - to avoid biased AI predictions,
- modeled - to correctly set up explainable or transparent AI models (see Explainable or Transparent AI),
- architected - to make its meta-model and underlying principles understandable and manageable for humans
- governed - to define a data lifecycle process with ownership and responsibilities, and mitigate risks and uncertainties
- accessible - by storing it in a cloud environment,
- regulated - and shared with regulatory institutions, to audit its usage
- protected - to avoid theft, abuse, and tampering.
Enhancing Customer Experience
Conference participants unanimously agreed that the main reason for employing AI in banking and insurance is to enhance customer experience, entailing greater availability by using chatbots and natural language processing.
Heard at the conference: “up to 55% of Q&A chat sessions are handled successfully by a chatbot”. Enhanced user experience also includes the development of new services, for instance by spotting opportunities in personal data, tailored to the needs of customers.
A particularly interesting take on customer experience was shared by BCGs Joerg Erlebach: Banks and insurance companies, he said, should act as a 'life’s concierge' and deliver concordant services to customers.
To personalize the customer experience, personal data need to be gathered. Notwithstanding the strict regulation on the collection and processing of personal data, customers seem willing to share large amounts of personal data in exchange for commodities like tailored customer service.
If customers are properly educated in what AI can mean to them, many benefits could be reaped in a way that is a win-win to companies and customers: “an educated customer is willing to pay more”.
Building explainable or transparent AI
Over the last decade, we have seen the emergence of ‘black box’ AI. Humans generally don’t understand how AI comes to its conclusions and predictions, one of the main reasons why the general public fears the technology.
Due to heavy regulations, maintained to avoid economical breakdown scenarios from the past, banks and insurance firms must be able to explain how they made their decisions (especially when things go wrong). Referring to predictions made by a “black box” AI is therefore not an option.
As early adopters of the digital transformation, banks and insurance companies prefer AI to be built on a ‘white box’ concept. If AI is ever going to be accepted by regulatory authorities, it should be transparent and explainable. Bankers and insurers should be able to explain any AI-based decision. There shouldn’t be any difference with data analyzed by humans.
Building explainable AI is far from trivial, due to the mathematical complexity at the heart of its algorithms. I think none of the sessions tackled this issue in a substantiated and satisfactory way.
As early adopters of the digital transformation, banks and insurance companies prefer AI to be built on a ‘white box’ concept. If AI is ever going to be accepted by regulatory authorities, it should be transparent and explainable.
Benjamin De Leeuw
The following solutions were suggested:
#1 Use open source AI algorithms
Open-source algorithms are developed from a community perspective and do not have a specific business goal in mind. They solely intend to implement the most up-to-date state of scientific research on AI and are generally not biased by commercial drivers. If needed, the mathematics underlying open-source AI libraries can be analyzed and backtracked by regulatory instances up to any level of detail.
#2 Learn to understand what are valid data sources
Data scientists should be trained in preprocessing and modeling data that is fed to an AI in such a way that the data displays statistical relevance (based on experience and lessons learned from the past) and biased conclusions can be avoided or corrected.
A recent example of a biased AI conclusion is the prediction of ideal job applicant profiles by Amazon. The company used an AI that was fed historical data on the success and retention rates of applicants from the past. Since the larger part of these applicants were male subjects, the AI concluded that the ideal candidate for the Amazon workforce would be male. This result is biased because the rather arbitrary historical consequences of a regional or company culture are, or should be, on a humanistic and logical ground, irrelevant when scanning what kind of applicants are fit for the job.
#3 Install responsibility by default
Secure data is the kingpin of company processes and strategy, obfuscated or encrypted when personal data e.g. is shared with other parties. Transparent communication on which data is gathered for what reason, who is responsible for it, and how it will be used, can show a willingness to the general public and to regulation authorities specifically, that data is handled responsibly.
#4 Valorize old data models
Old data models do not become irrelevant when introducing AI. These old models frequently contain insights and business acumen from over decades. Instead of throwing them away, incorporate them into the AI data models. Arguably, this view conflicts with the statement that companies should not improve their processes, but rather build them anew from the ground up.
#5 Bring human and AI together
If the humans working with AI do not understand it, ‘consumers’ will not trust AI-generated or produced outcome, especially when those people are part of a regulatory instance.
#6 Cooperate closely with regulatory instances
Regulation can drive innovation and enhance understanding of what is going on inside AI technology. Though conference participants did not elaborate on this topic, the innovation at stake could imply the ability to display the evolution of an AI through reports or user interfaces in a comprehensible fashion.
It seems that there is not really anything else that can be done at this moment. Arguably, regulatory instances are willing to accept these solutions, as testified by some of the speakers.
Consider, however, this catchphrase I heard during one of the talks:
“Not all black-box AI is necessarily unreasonable, and not all white-box AI is necessarily reasonable”.
Next installment
- Ethics, Responsibility, Regulation, and Protection
- Succesful AI Deployment
- Bank versus Fintech
Related content
-
Article
5 essential elements for a credible sustainability report
-
Blog
Embedding Third-party Risk Management into strategic resilience
-
Reference case
Setting the standard for CSRD at a leading European retailer
-
Article
Beyond complexity: Driving sustainable change at a large Belgian insurer with TriFinance
-
Blog
Striking results from our 2025 Finance Transformation Survey
-
Blog
Growth Story: Jorn Hillaert – Building change through people and co-creation